Le Pronostic en OR
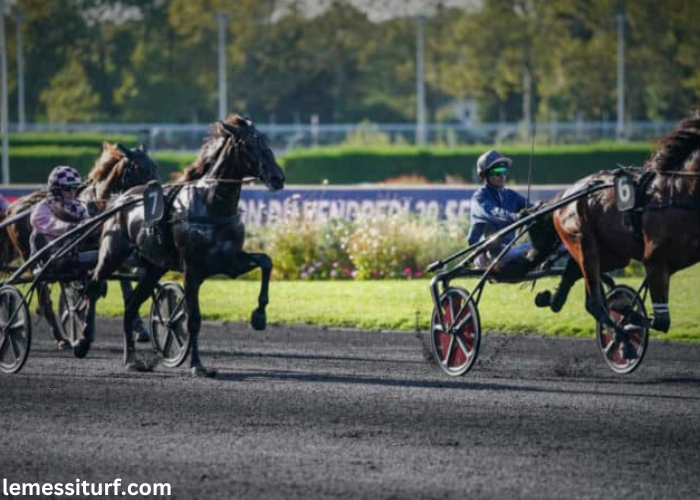
In a world characterized by uncertainty and rapid change, the ability to make accurate predictions has become a coveted skill. Le Pronostic en OR, or “The Prediction in Gold,” encapsulates this idea, emphasizing the value of reliable forecasts across various fields, from finance to sports and even personal decisions. This article explores the nuances of predictive forecasting, delving into the methodologies, the psychology behind predictions, and the implications of accurate forecasting in our daily lives.
Understanding Predictive Forecasting
Predictive forecasting involves estimating future events based on historical data, trends, and analytical techniques. It combines both art and science, requiring a deep understanding of the subject matter, analytical skills, and sometimes even a touch of intuition. The practice is not limited to one discipline; it spans across economics, business, weather forecasting, sports analytics, and even social sciences.
At its core, predictive forecasting aims to provide insights that help individuals and organizations make informed decisions. The implications of accurate predictions can be profound, influencing everything from investment strategies to resource allocation and policy-making. In a business context, for example, accurate sales forecasts can determine inventory levels, pricing strategies, and marketing efforts.
The Methodologies Behind Forecasting
There are various methodologies employed in predictive forecasting, each with its own strengths and limitations. Quantitative methods rely heavily on numerical data and statistical techniques to make predictions. Common quantitative methods include regression analysis, time series analysis, and machine learning algorithms. These techniques help analysts identify patterns, trends, and correlations that may not be immediately apparent.
Regression analysis, for instance, examines the relationship between variables to predict outcomes. If a company wants to forecast its sales based on advertising expenditure, regression analysis can provide insights into how changes in one variable affect another. Time series analysis, on the other hand, focuses on historical data points over time to identify seasonal patterns and trends.
Qualitative methods, in contrast, rely on expert judgment and subjective analysis. These methods are particularly useful when historical data is sparse or when predicting complex phenomena where human behavior plays a significant role. Expert opinions, focus groups, and scenario planning are examples of qualitative techniques. Combining both quantitative and qualitative approaches often yields the most accurate forecasts.
The Role of Data in Predictive Forecasting
Data serves as the foundation of predictive forecasting. The quality and quantity of data significantly impact the reliability of predictions. As technology has advanced, the availability of data has exploded, leading to new opportunities and challenges. Big data, encompassing vast datasets from various sources, allows analysts to refine their predictions and uncover hidden patterns.
However, with the abundance of data comes the challenge of data quality. Inaccurate or incomplete data can lead to misleading forecasts, highlighting the importance of rigorous data validation and cleaning processes. Moreover, understanding the context in which data is collected is essential. Factors such as data bias, external influences, and changing conditions can affect the accuracy of predictions.
Additionally, the rise of artificial intelligence and machine learning has revolutionized the field of predictive analytics. These technologies can process vast amounts of data in real-time, identifying trends and making predictions with remarkable speed and accuracy. While these advancements hold great promise, they also raise ethical concerns regarding data privacy and the potential for algorithmic bias.
The Psychology of Prediction
The act of predicting is as much psychological as it is analytical. Cognitive biases can significantly influence our ability to make accurate forecasts. For instance, confirmation bias leads individuals to favor information that supports their existing beliefs while disregarding contradictory evidence. This bias can distort predictions and result in poor decision-making.
Moreover, overconfidence is a common psychological pitfall. Individuals often overestimate their knowledge and predictive abilities, leading to reckless decisions based on inflated confidence. Recognizing these cognitive biases is crucial for improving forecasting accuracy. By adopting a more critical and reflective mindset, forecasters can mitigate the impact of these biases and make better-informed predictions.
Additionally, emotional factors can play a role in predictive forecasting. Anxiety about future outcomes can lead to overly conservative predictions, while optimism may result in unrealistic forecasts. Striking a balance between rational analysis and emotional awareness is essential for effective prediction.
Applications of Predictive Forecasting
The applications of predictive forecasting are vast and varied, impacting numerous sectors and industries. In finance, predictive models are used to forecast stock prices, interest rates, and market trends. Investors rely on these predictions to make informed decisions about buying, selling, and holding assets.
In the healthcare sector, predictive analytics plays a critical role in patient care and resource management. By analyzing patient data, healthcare providers can anticipate patient needs, improve treatment plans, and enhance overall operational efficiency. Predictive models can help identify potential health risks, enabling proactive measures that can lead to better patient outcomes.
Similarly, in marketing, businesses utilize predictive analytics to understand consumer behavior, optimize advertising campaigns, and improve customer engagement. By analyzing past purchasing patterns and demographic data, companies can tailor their marketing strategies to target specific audiences effectively.
Sports analytics is another area where predictive forecasting has gained prominence. Teams use data-driven insights to assess player performance, evaluate game strategies, and enhance overall team dynamics. Predictive models can even help identify potential injuries, allowing teams to make informed decisions about player health and safety.
The Challenges of Predictive Forecasting
Despite its potential benefits, predictive forecasting is not without challenges. The inherent uncertainty of future events means that no prediction can be guaranteed to be accurate. External factors, such as economic downturns, natural disasters, and geopolitical changes, can disrupt even the most meticulously crafted forecasts.
Additionally, the complexity of human behavior poses a significant challenge. People often act unpredictably, making it difficult to model their actions accurately. This complexity is particularly evident in fields such as economics and social sciences, where human emotions and motivations play a significant role.
Furthermore, the fast-paced nature of modern life can render forecasts obsolete almost immediately. As conditions change rapidly, predictions may become outdated, necessitating continuous monitoring and adjustment. Organizations must remain agile and willing to adapt their strategies in response to evolving circumstances.
The Future of Predictive Forecasting
As we move further into the digital age, the future of predictive forecasting appears promising yet complex. The integration of artificial intelligence and machine learning is likely to continue reshaping the landscape of predictive analytics. These technologies can analyze data at an unprecedented scale and speed, enabling more accurate and timely predictions.
Moreover, the increasing emphasis on data ethics and responsible AI is shaping the future of predictive forecasting. As organizations harness the power of data, they must also consider the ethical implications of their predictive models. Ensuring data privacy, minimizing bias, and fostering transparency will be critical for maintaining public trust.
Additionally, the growing importance of interdisciplinary collaboration cannot be overstated. Predictive forecasting often requires expertise from various fields, including statistics, computer science, psychology, and domain-specific knowledge. By fostering collaboration across disciplines, organizations can develop more comprehensive and effective predictive models.
Conclusion
Le Pronostic en OR embodies the intricate dance between art and science in the realm of predictive forecasting. As we navigate an increasingly complex world, the ability to make accurate predictions has never been more valuable. By understanding the methodologies, data-driven insights, and psychological factors that influence forecasting, individuals and organizations can make informed decisions that drive success.
As technology continues to advance and data becomes more accessible, the future of predictive forecasting holds great promise. However, it is essential to remain vigilant in addressing the challenges and ethical considerations that accompany these advancements. Ultimately, the journey of predictive forecasting is one of continuous learning and adaptation, offering a powerful tool for navigating the uncertainties of life and achieving our goals.